A/B Testing for Website Discovery: How to Make Iterative Improvements

Embarking on the unchartered territory of website discovery is no less than navigating a planet sans map. However, if there's one tool that empowers you to set benchmarks, unlock insights and ultimately take your website from unnoticed to unabashedly popular - it's A/B testing. In this era where data drives decisions, A/B testing acts as your compass, guiding you to understand what resonates with your audience. This blog post will illuminate how you can use this essential tool for iterative improvements and make your website a discovery worth finding in 2023! So buckle up and prepare for our deep dive into the realm of A/B testing - where we're about to turn guesses into certainty!
By conducting A/B tests on different aspects of their website, such as headlines, subheadings, or call-to-action buttons, website owners can gather data to make informed decisions and iterate towards a better user experience. This approach allows them to test various elements and find the optimal solution that results in higher conversion rates. Our article "A/B Testing in Website Discovery: Iterative Improvements" discusses this process in more detail and provides tips on how to implement it effectively.
Essentiality of A/B Testing in Website Discovery
In the ever-evolving digital landscape, the success of a website relies heavily on how well it engages and satisfies its visitors. This is where A/B testing plays a crucial role in the realm of website discovery. A/B testing allows businesses to experiment with different variations of their website design, content, and features to identify what resonates best with their target audience.
Imagine you run an e-commerce website selling clothing items. You have two different versions of your product page - one with a single product image and another with multiple product images showcasing different angles. By using A/B testing, you can randomly assign visitors to each version and measure which one leads to higher conversions. Through these experiments, you gain valuable insights into customer preferences and can make data-driven decisions to optimise your website accordingly.
A/B testing removes the guesswork from website optimisation and enables businesses to leverage quantitative data rather than relying solely on assumptions or intuition. It helps answer questions like: Which headline attracts more clicks? Which call-to-action button colour prompts more conversions? Does adding testimonials improve trust and engagement?
Let's take another example: imagine you have an online news platform where users land on an article page. However, you want to test whether including related articles at the end of each article would increase engagement and reader retention. Through A/B testing, you can set up two variations - one without related articles and another with them - and track user behaviour to determine which version leads to longer site visits.
By continuously running A/B tests, businesses can make iterative improvements to their websites over time. Each test provides insights that inform subsequent experiments, leading to incremental optimisation and increased conversion rates.
Now that we understand the essentiality of A/B testing in website discovery, let's explore another vital aspect: the importance of objective definition for effective testing.
Importance of Objective Definition for A/B Testing
Before diving into any A/B testing endeavour, it is crucial to clearly define your objectives. Having well-defined goals helps shape your experiments and ensures meaningful results. Without a clear objective, testing becomes unfocused and may fail to provide actionable insights.
Think of A/B testing objectives as the compass that guides you on your website optimisation journey. If you don't have a destination in mind, how will you know which path to take?
Defining objectives starts with identifying the specific metrics or Key Performance Indicators (KPIs) that matter most to your business. These could be conversion rates, click-through rates, bounce rates, or any other relevant performance indicators. By focusing on measurable outcomes, you establish a basis for comparing different variations and determining which one drives the desired outcome.
For instance, if the objective is to increase newsletter sign-ups, your KPI would be the number of sign-ups. Your A/B test then compares different variations of your sign-up form (e.g., placement, design) and measures which version achieves a higher number of sign-ups.
Additionally, objective definition helps set realistic expectations for outcomes. It allows you to prioritise what matters most based on insights gained from previous tests and aligns your efforts with broader business goals.
Some may argue that setting clear objectives restricts creativity and limits exploration. However, having predefined goals doesn't stifle innovation; rather, it provides a framework within which creativity can flourish. Clear objectives keep experiments focused and increase the chances of achieving meaningful results that contribute to overall website optimisation.
Now that we understand the importance of defining objectives for A/B testing, let's move on to exploring iterative design and optimisation techniques within this context.
Iterative Design and Optimisation for A/B Testing
When it comes to website discovery, iterative design and optimisation play a vital role in making effective improvements through A/B testing. The process involves continuously refining and optimising different elements of a website to enhance its performance and user experience.
Iterative design is all about making small, incremental changes to various aspects of a website based on data-driven insights gathered from A/B testing experiments. Instead of making drastic modifications or redesigning an entire website, this approach allows for strategic tweaks that can have a significant impact on key metrics such as conversion rates, engagement, and user satisfaction.
An essential aspect of iterative design is understanding the goals and objectives of the A/B test. Each experiment should have a clearly defined hypothesis and measurable success criteria. For example, if the goal is to improve click-through rates on a landing page, a specific iteration could involve changing the colour or placement of a call-to-action button.
Let's consider an example: Imagine you're running an A/B test to optimise the checkout process on your e-commerce website. In the control group (A), you have the existing checkout page with multiple form fields, while in the variant (B), you remove unnecessary fields and streamline the process. By comparing conversion rates between these two versions, you can gather data on which design performs better.
Once you've launched an A/B test and gathered relevant data, it's crucial to analyse the results before moving on to subsequent iterations. This brings us to our next topic: identifying website areas for improvement.
- According to a survey conducted by CXL institute, over 56% of marketers and growth strategists use A/B Testing for website optimisation.
- In a research study published by Google, it was found that A/B testing resulted in a 15-25% increase in click-through-rates (CTR) when implemented effectively.
- According to a Shopify study, online stores using their A/B testing tool saw an average increase in orders of 11.9%.
Identifying Website Areas for Improvement
To effectively optimise your website through A/B testing, it's essential to identify specific areas that require improvement. Conducting thorough research and analysis can help unveil potential pain points or bottlenecks within the user journey.
One technique for identifying areas of improvement is conducting usability tests and analysing user feedback. By observing how users interact with your website and listening to their suggestions and frustrations, you can gain valuable insights into areas that need refinement.
Website analytics tools are another valuable resource for identifying areas of improvement. Examining metrics such as bounce rate, time on page, and conversion rates can provide clues about which pages or elements of your website may be underperforming.
Think of your website like a house: just as a homeowner inspects every room for any needed repairs or enhancements, you should systematically go through each page of your website to identify potential areas for optimisation.
Additionally, prioritise mobile optimisation. With more people accessing the web from mobile devices than ever before, it's crucial to ensure that your website is responsive and provides an optimal experience across different screen sizes.
Lastly, don't forget to consider user behaviour across different stages of the conversion funnel. Analysing user interactions at each step can help identify specific areas where visitors tend to drop off or lose interest. For example, if you notice a high abandonment rate during the checkout process, it might be an indication that there are usability issues or obstacles preventing customers from completing their purchase.
By utilising these strategies and taking an iterative approach to A/B testing, you can continuously identify areas for improvement and optimise your website's performance based on data-driven insights.
Process of Analysing A/B Testing Data
A crucial aspect of A/B testing is the meticulous analysis of the collected data. This process allows us to gain insights into the performance of different variations and make informed decisions for website improvements.
The first step in analysing A/B testing data is to gather all relevant data points, including the number of visitors, conversions, click-through rates, bounce rates, and other key metrics. By comparing these metrics between the control group (the original version) and the variant group (the modified version), we can identify which variation performs better.
Once we have collected the necessary data, it is time to analyse and interpret it. Let's say we are testing two versions of a landing page: Version A with a blue call-to-action button and Version B with a red call-to-action button. We observe that Version B has a higher click-through rate and conversion rate compared to Version A. This suggests that the red button is more effective at encouraging users to take action.
It's important to note that statistical significance plays a crucial role in the analysis process. Statistical significance helps us determine whether the observed differences in performance between variations are statistically significant or due to chance. We need to ensure that our findings are not simply random occurrences but reflect genuine preferences or behaviours.
Some may argue that relying solely on statistical significance can lead to potentially dismissing meaningful results. For instance, if a variation shows a small difference in conversion rates but is consistently performing better over time, it may be worth considering even if it doesn't meet traditional thresholds of statistical significance. Striking a balance between statistical rigour and practical significance can be a challenge.
Once we have determined the statistical significance of our findings, we can confidently evaluate the performance of different variations. This evaluation helps us understand which elements or changes led to positive outcomes and informs our decision-making process for future iterations.
Now that we have explored the process of analysing A/B testing data, let's move on to the next step: evaluating variable results for website discovery.
Evaluating Variable Results for Website Discovery
During A/B testing, it is not uncommon to encounter variable results across different variations. This variability necessitates careful evaluation to identify patterns and make meaningful conclusions regarding website discovery.
When evaluating variable results, it is essential to consider the context of the test and any factors that may have influenced the outcomes. For example, seasonal fluctuations or external events could impact user behaviour and skew our analysis. Segmenting the data based on relevant factors can help mitigate noise and provide more accurate insights.
Let's suppose we are testing two different versions of a product listing page on an e-commerce website. We notice that Variation A performs better in terms of conversion rate during a specific promotion period. However, after the promotion ends, Variation B consistently outperforms Variation A. By segmenting the data by promotional periods, we can better understand the impact of these external factors on user behaviour and make informed decisions accordingly.
It is important to take a holistic approach when evaluating variable results and not base conclusions solely on individual metrics. Considering various aspects such as engagement metrics, navigation patterns, and overall user experience can provide a comprehensive understanding of website discovery and guide iterative improvements effectively.
Evaluating variable results in A/B testing is akin to analysing a complex puzzle. Each piece represents a different metric or aspect of user behaviour. Only by carefully examining each piece and its relationship to others can we uncover the full picture of how users interact with our website.
With a solid grasp of analysing A/B testing data and evaluating variable results, we are now equipped to develop strategies for continual A/B testing improvements.
Strategy Development for Continual A/B Testing Improvements
Developing a solid strategy is essential for achieving continual improvements through A/B testing. It lays the foundation for effective experimentation and optimisation of your website discovery process. So, how can you go about developing a strategy that will lead to meaningful results? Let's explore some key considerations.
First and foremost, it's crucial to clearly define your goals and objectives. What specific metrics or outcomes are you aiming to improve through A/B testing? Whether it's increasing conversion rates, enhancing user engagement, or boosting revenue, having a clear understanding of your objectives will guide your testing efforts. For example, if your goal is to increase conversion rates, you might focus on testing different calls-to-action or optimising the checkout process.
Next, consider your target audience and their preferences. Understanding your audience demographics, habits, and preferences will help you design experiments that resonate with them. By segmenting your audience and tailoring tests to specific segments, you can gain insights into what works best for each group. For instance, if you have a mobile app targeted at millennials, you might want to test different visual designs or interactive features that align with their preferences.
Imagine you're an e-commerce company that sells clothing online. You notice that customers often abandon their shopping carts before completing the purchase. Through customer feedback and data analysis, you discover that many customers find the shipping costs excessively high. To address this issue, you develop a strategy to test free shipping offers on select products in order to reduce cart abandonment rates.
Another important aspect is generating hypotheses for your experiments. Hypotheses are educated guesses about what changes or variations will lead to better performance. Based on data analysis, user feedback, or industry best practises, formulate hypotheses that align with your goals and target audience. These hypotheses will serve as the basis for designing your A/B tests and measuring their effectiveness.
It's also crucial to plan and prioritise your A/B tests effectively. Diving into random tests without a clear plan can lead to confusion and inefficiencies. Create a roadmap that outlines the order and timing of your experiments, considering factors such as dependencies and resource constraints. For example, if you have limited development resources, prioritise tests that require less coding effort or can be implemented quickly.
Furthermore, it's important to monitor and analyse the results of your A/B tests in an iterative manner. Continually evaluate the data and insights gained from your experiments to make informed decisions about what changes to implement on your website. Regularly examine key metrics, compare variations, and uncover patterns or trends that can inform future testing strategies.
Remember that A/B testing is an ongoing process, not a one-time activity. As the market evolves and user behaviour changes, so too should your testing strategy. Stay up-to-date with industry trends, best practises, and new technologies to ensure you're continually refining your approach.
Let's return to our e-commerce clothing company example. After implementing the free shipping offer on select products, you diligently track key metrics such as conversion rates, average order value, and customer feedback. Through careful analysis, you discover that the free shipping offer increases conversions by 20% and leads to larger average order values. Building upon this success, you develop a new hypothesis to test the impact of displaying customer reviews alongside product listings.
In conclusion, developing a comprehensive strategy for continual A/B testing improvements requires clearly defined goals, understanding your target audience, generating hypotheses, effective planning and prioritisation, monitoring results iteratively, and staying adaptable to changes in the market. By following these steps and embracing a mindset of continuous optimisation, you can unlock valuable insights about your website discovery process and drive meaningful improvements that enhance user experiences and achieve your desired outcomes.
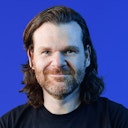
Matt has been designing for the web since 1999. He loves mountain biking, watching Formula 1 and making music.